Unlock the Secrets of Data Sourcing: What Is Data Sourcing
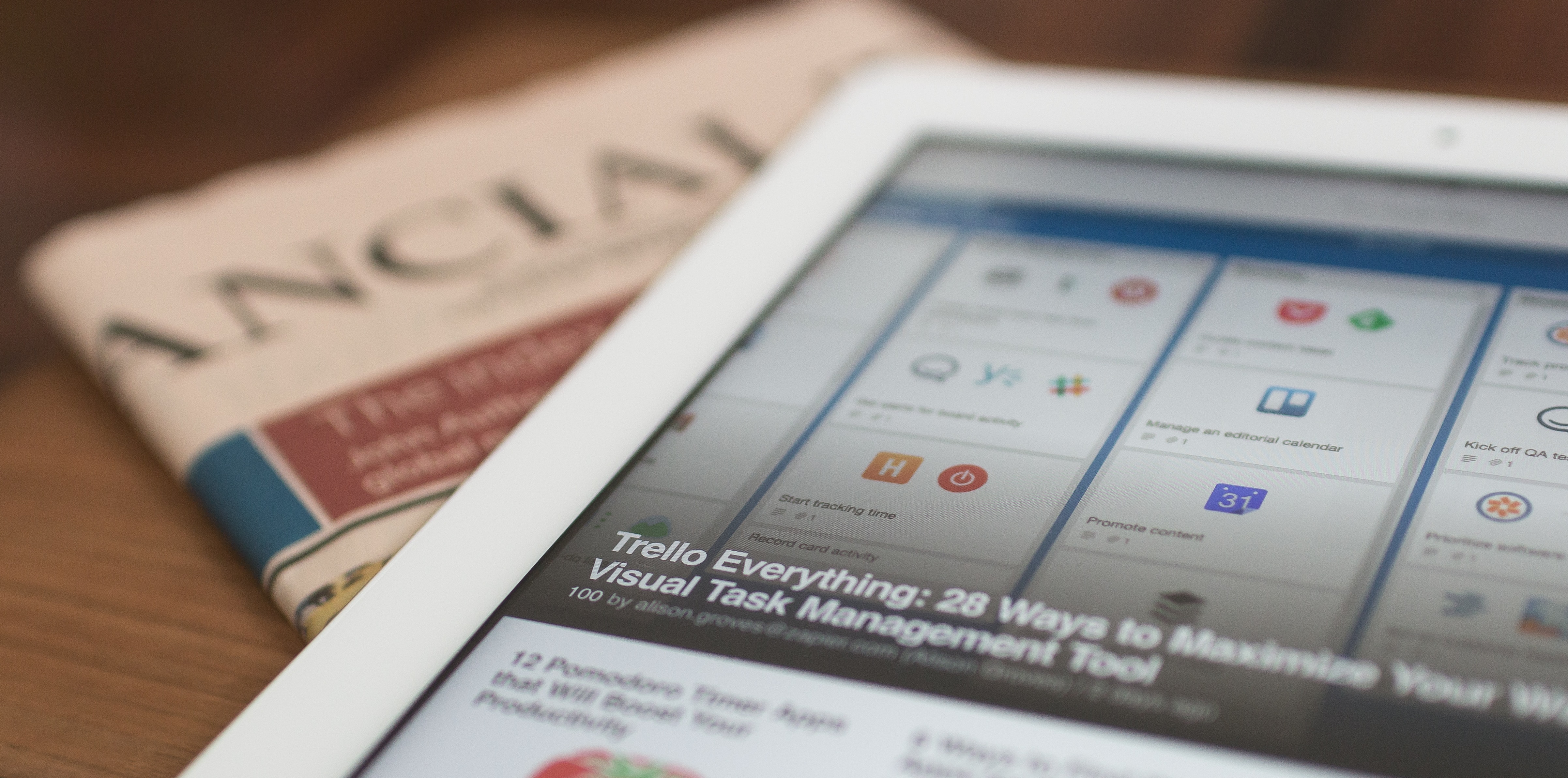
Data sourcing is a critical process for data scientists and analysts, as it enables them to access the most relevant datasets for their projects. It involves searching through large amounts of information from multiple machine data sources in order to identify the best available data sets that can be used effectively. Data sourcing necessitates an awareness of the sources where distinct data types can be located, how to gain access to them, and what approaches should be taken when collecting them. This article will discuss what is data sourcing, how to source it efficiently and safely, potential challenges encountered during this process, as well as some best practices that ensure successful results every time.
What is Data Sourcing?
Data sourcing is the process of collecting and organizing data from various sources. It involves gathering, analyzing, and interpreting information from both internal and external data sources to inform decisions or create insights. Data sourcing types can be employed for a range of objectives, like marketing initiatives, client stratification, product creation, hazard control, or fraud recognition.
Data sourcing can be described as any approach that allows an organization to obtain the data necessary for informed decisions. This could include anything from web scraping tools and APIs to customer surveys and interviews. The types of data sources available depend on the specific needs of each organization but typically include public records, social media posts, market research studies, customer feedback surveys, etc.
By combining multiple data sources, organizations can gain a comprehensive understanding of their target audience and uncover patterns that could not have been seen with just one type alone, thus leading to new opportunities for growth. Additionally, leveraging different kinds of data helps companies identify patterns that may not have been visible when looking at just one type alone, which can lead to new opportunities for growth or improved processes within the business itself. Finally, having access to reliable datasets also reduces time spent manually researching topics, making it easier for teams to focus on more important tasks such as developing strategies based on their findings.
Data sourcing is an important process for data scientists and analysts to acquire the necessary data needed for their projects. Realizing the significance of acquiring suitable data, it is essential to know how to acquire it proficiently.
How to Source Data?
Identifying the Right Sources
When sourcing data, it’s important to find reliable sources of information. This means researching a variety of different sources and determining which ones are most trustworthy and accurate. For example, if you’re looking for statistics about a certain industry or market, you might consider consulting trade journals or official government websites. Connecting with people in your sector can provide invaluable information and understanding.
Gathering and Organizing the Data
Once you have identified quality sources of data, it is essential to gather all pertinent information from these sources and consolidate it into one location for orderly analysis. This necessitates careful preparation and organization - think "measure twice, cut once." Automation tools such as web scraping software can expedite this procedure by rapidly accumulating substantial amounts of data from multiple online resources with minimal human input.
Analyzing and Interpreting the Data
The final step in effective data sourcing is analyzing and interpreting what has been collected. This involves breaking down complex datasets into meaningful chunks that allow you to draw insights from them – essentially turning raw numbers into actionable intelligence. It also requires critical thinking skills to make sure that any conclusions drawn are based on sound logic rather than unfounded assumptions or biases. With enough practice (and maybe a few sleepless nights) anyone can become adept at understanding how various pieces fit together within larger datasets, making informed decisions easier than ever before.
Obtaining quality, trustworthy data is a key element of data science and can be challenging; however, with the correct strategies in place managing large amounts of information becomes more manageable. Nevertheless, deploying the proper approaches can make managing copious amounts of data much more feasible. Next up we will discuss some common data sourcing challenges associated with sourcing data.
Key Takeaway: Data sourcing requires a methodical approach, from identifying reliable sources and gathering data to analyzing and interpreting it. Automation tools can help streamline the process by quickly collecting large amounts of information with minimal effort. Ultimately, successful data sourcing is about turning raw numbers into actionable intelligence through careful planning and critical thinking.
Challenges in Data Sourcing
Data sourcing is an essential part of data science and analysis. It involves the collection, organization, and evaluation of data from multiple sources to produce meaningful insights. Finding high data quality that is trustworthy and precise can be a difficult task.
Uncovering trustworthy data sources can be a difficult task for any data scientist, requiring the ability to identify reliable datasets and evaluate if websites are dependable enough to draw from. This means understanding where to look for relevant datasets or determining which websites are trustworthy enough to use as a source for research. Data scientists must assess if the data they acquire is current and includes all necessary details before utilizing it in any endeavor or study. Additionally, they need to ensure that the sourced data is not biased towards any particular viewpoint or outcome by cross-checking facts with other resources if needed.
Ensuring Accuracy and Reliability of the Data:
Once a suitable source has been identified, it’s important to check its accuracy and reliability before using it in any project or analysis. This involves examining the data closely for errors such as typos or inconsistencies between different pieces of information within a dataset - something that can easily be overlooked but can have serious implications on results if left unchecked. Furthermore, verifying that each piece of information comes from an authoritative source will help guarantee its accuracy too - this could mean double checking references against published studies/research papers, etc., depending on what type of dataset you’re working with.
Handling large datasets can be an intimidating task due to the time it takes to arrange them into usable parts so that meaningful results may be obtained. To make things easier, many professionals use software tools like Tableau or Power BI which allow users to quickly visualize their datasets without having to manually sort through hundreds (or even thousands) of lines worth of information first. These platforms come equipped with built-in features such as filtering options which makes searching through large amounts of info much more efficient than doing so manually would ever be possible too.
Data sourcing is a complex process that requires careful planning and execution. With the right strategies, however, data sourced effectively can meet any organization's needs. To ensure a successful data collection process, it is important to understand best practices for effective data sourcing.
Key Takeaway: Obtaining quality and dependable data is a key part of data science, yet can be challenging. Before utilizing any source for a project or analysis, it is wise to verify its accuracy and dependability through software such as Tableau or Power BI that can facilitate the visualization of sizable datasets.
Best Practices for Effective Data Sourcing
Accurately obtaining data is a critical component of any data science or analytics venture. In order to ensure that the data used in a project is accurate and reliable, it’s essential to use best practices when collecting and storing information. Establishing clear goals and objectives is the first step toward effective data sourcing. Knowing the kind of data that is necessary, how it will be employed, and where it should originate from are all essential to guaranteeing a successful project.
Using automation tools for efficiency can also help streamline the process of gathering large amounts of information quickly and accurately. Automation tools such as web crawlers can save time by automatically harvesting relevant content from websites without manual intervention. Moreover, these tools are capable of harvesting data more thoroughly and expeditiously than if done manually.
Creating and preserving exactness among multiple sources over extended periods necessitates a structured system for collecting and archiving data. By developing standards for categorizing different types of information – such as timestamps, locations, or file formats – organizations can create consistency throughout their datasets which makes them easier to analyze later on down the line. It’s also beneficial to keep track of changes made along the way so that all stakeholders involved have access to updated versions if needed in future projects or reports.
Ultimately, following best practices when sourcing data helps organizations maximize their investments in their projects while minimizing potential risks associated with inaccurate or unreliable datasets. By taking proactive steps such as establishing clear goals and objectives upfront; utilizing automation tools; adopting a systematic approach; companies can ensure they have access to good data sources that are up-to-date with current trends in their industry so they don't get left behind.
Key Takeaway: Data acquisition is a must for any fruitful data science or analytics initiative, and it's best to employ good practices in order to guarantee accuracy and dependability. Automation tools such as web crawlers can help streamline the process by quickly harvesting large amounts of information with precision while developing standards for categorizing different types of data will create consistency throughout datasets over long periods of time. By following these steps proactively, organizations can maximize their investments in projects while minimizing risks associated with inaccurate sources.
FAQs in Relation to What is Data Sourcing
What is data sourcing?
This includes collecting information from databases, websites, APIs (application programming interfaces), and other online resources. Data sourcing can also involve extracting data manually or using automated tools such as web scraping to collect unstructured data. Businesses can gain advantageous knowledge by utilizing various data acquisition techniques that would be inaccessible with conventional methods.
What is an example of a data source?
A data source is any location or repository from which data can be obtained. Examples of file data sources include web APIs, databases, text files, CSV files, spreadsheets, and other structured documents such as XML and JSON. Data sources may also come from online services like social media platforms or external third-party applications that provide access to information. Furthermore, various tools can be employed to draw out unstructured data, for instance, web scraping technology which allows users to obtain a large quantity of pertinent information quickly and effortlessly.
1. Web APIs:
Web APIs are a great source of data for web extraction projects as they provide access to structured and unstructured data from websites, databases, and other sources in an organized manner.
2. HTMLs:
By leveraging HTML scraping, it is possible to gather specific pieces of information or entire datasets from web pages. It can be used to extract specific pieces of information or entire datasets from web pages.
3. Databases:
Databases are a popular source of data, thanks to their capacity for keeping vast amounts of structured information in an orderly fashion that can be readily examined and analyzed.
4. Social Media Data:
Social media platforms like Twitter, Facebook, Instagram, etc., offer up valuable insights into consumer behavior which can be leveraged through web extraction techniques such as API calls or text mining algorithms applied directly on social media posts/comments/reviews, etc.
5 File Downloads:
Files can be easily acquired from the web with specialized tools which enable rapid downloading of extensive amounts of unprocessed information without having to manually search each page or create database inquiries.
What are the types of data sources?
Data sources can be divided into two main categories: structured and unstructured. Structured data sources refer to those which possess a predetermined format, such as databases, spreadsheets or other forms of organized storage. Unstructured data sources include webpages, emails, images, videos and audio files which do not have any specific structure or organization. Data scientists and analysts use various techniques to extract meaningful information from these different types of data sources in order to gain insights for their projects.
Conclusion
With careful planning and thoughtful execution, it's possible to source quality datasets that enable meaningful insights into complex problems. By following best practices such as researching potential sources thoroughly before beginning extraction work or validating accuracy after acquisition, organizations can ensure their success in collecting valuable data sets through effective data-sourcing processes.
Let us help you unlock the potential of your data with our comprehensive web data extraction solutions. Our team of experts can provide tailored strategies to ensure that you get the most out of your online resources.
Data sourcing is a critical process for data scientists and analysts, as it enables them to access the most relevant datasets for their projects. It involves searching through large amounts of information from multiple machine data sources in order to identify the best available data sets that can be used effectively. Data sourcing necessitates an awareness of the sources where distinct data types can be located, how to gain access to them, and what approaches should be taken when collecting them. This article will discuss what is data sourcing, how to source it efficiently and safely, potential challenges encountered during this process, as well as some best practices that ensure successful results every time.
What is Data Sourcing?
Data sourcing is the process of collecting and organizing data from various sources. It involves gathering, analyzing, and interpreting information from both internal and external data sources to inform decisions or create insights. Data sourcing types can be employed for a range of objectives, like marketing initiatives, client stratification, product creation, hazard control, or fraud recognition.
Data sourcing can be described as any approach that allows an organization to obtain the data necessary for informed decisions. This could include anything from web scraping tools and APIs to customer surveys and interviews. The types of data sources available depend on the specific needs of each organization but typically include public records, social media posts, market research studies, customer feedback surveys, etc.
By combining multiple data sources, organizations can gain a comprehensive understanding of their target audience and uncover patterns that could not have been seen with just one type alone, thus leading to new opportunities for growth. Additionally, leveraging different kinds of data helps companies identify patterns that may not have been visible when looking at just one type alone, which can lead to new opportunities for growth or improved processes within the business itself. Finally, having access to reliable datasets also reduces time spent manually researching topics, making it easier for teams to focus on more important tasks such as developing strategies based on their findings.
Data sourcing is an important process for data scientists and analysts to acquire the necessary data needed for their projects. Realizing the significance of acquiring suitable data, it is essential to know how to acquire it proficiently.
How to Source Data?
Identifying the Right Sources
When sourcing data, it’s important to find reliable sources of information. This means researching a variety of different sources and determining which ones are most trustworthy and accurate. For example, if you’re looking for statistics about a certain industry or market, you might consider consulting trade journals or official government websites. Connecting with people in your sector can provide invaluable information and understanding.
Gathering and Organizing the Data
Once you have identified quality sources of data, it is essential to gather all pertinent information from these sources and consolidate it into one location for orderly analysis. This necessitates careful preparation and organization - think "measure twice, cut once." Automation tools such as web scraping software can expedite this procedure by rapidly accumulating substantial amounts of data from multiple online resources with minimal human input.
Analyzing and Interpreting the Data
The final step in effective data sourcing is analyzing and interpreting what has been collected. This involves breaking down complex datasets into meaningful chunks that allow you to draw insights from them – essentially turning raw numbers into actionable intelligence. It also requires critical thinking skills to make sure that any conclusions drawn are based on sound logic rather than unfounded assumptions or biases. With enough practice (and maybe a few sleepless nights) anyone can become adept at understanding how various pieces fit together within larger datasets, making informed decisions easier than ever before.
Obtaining quality, trustworthy data is a key element of data science and can be challenging; however, with the correct strategies in place managing large amounts of information becomes more manageable. Nevertheless, deploying the proper approaches can make managing copious amounts of data much more feasible. Next up we will discuss some common data sourcing challenges associated with sourcing data.
Key Takeaway: Data sourcing requires a methodical approach, from identifying reliable sources and gathering data to analyzing and interpreting it. Automation tools can help streamline the process by quickly collecting large amounts of information with minimal effort. Ultimately, successful data sourcing is about turning raw numbers into actionable intelligence through careful planning and critical thinking.
Challenges in Data Sourcing
Data sourcing is an essential part of data science and analysis. It involves the collection, organization, and evaluation of data from multiple sources to produce meaningful insights. Finding high data quality that is trustworthy and precise can be a difficult task.
Uncovering trustworthy data sources can be a difficult task for any data scientist, requiring the ability to identify reliable datasets and evaluate if websites are dependable enough to draw from. This means understanding where to look for relevant datasets or determining which websites are trustworthy enough to use as a source for research. Data scientists must assess if the data they acquire is current and includes all necessary details before utilizing it in any endeavor or study. Additionally, they need to ensure that the sourced data is not biased towards any particular viewpoint or outcome by cross-checking facts with other resources if needed.
Ensuring Accuracy and Reliability of the Data:
Once a suitable source has been identified, it’s important to check its accuracy and reliability before using it in any project or analysis. This involves examining the data closely for errors such as typos or inconsistencies between different pieces of information within a dataset - something that can easily be overlooked but can have serious implications on results if left unchecked. Furthermore, verifying that each piece of information comes from an authoritative source will help guarantee its accuracy too - this could mean double checking references against published studies/research papers, etc., depending on what type of dataset you’re working with.
Handling large datasets can be an intimidating task due to the time it takes to arrange them into usable parts so that meaningful results may be obtained. To make things easier, many professionals use software tools like Tableau or Power BI which allow users to quickly visualize their datasets without having to manually sort through hundreds (or even thousands) of lines worth of information first. These platforms come equipped with built-in features such as filtering options which makes searching through large amounts of info much more efficient than doing so manually would ever be possible too.
Data sourcing is a complex process that requires careful planning and execution. With the right strategies, however, data sourced effectively can meet any organization's needs. To ensure a successful data collection process, it is important to understand best practices for effective data sourcing.
Key Takeaway: Obtaining quality and dependable data is a key part of data science, yet can be challenging. Before utilizing any source for a project or analysis, it is wise to verify its accuracy and dependability through software such as Tableau or Power BI that can facilitate the visualization of sizable datasets.
Best Practices for Effective Data Sourcing
Accurately obtaining data is a critical component of any data science or analytics venture. In order to ensure that the data used in a project is accurate and reliable, it’s essential to use best practices when collecting and storing information. Establishing clear goals and objectives is the first step toward effective data sourcing. Knowing the kind of data that is necessary, how it will be employed, and where it should originate from are all essential to guaranteeing a successful project.
Using automation tools for efficiency can also help streamline the process of gathering large amounts of information quickly and accurately. Automation tools such as web crawlers can save time by automatically harvesting relevant content from websites without manual intervention. Moreover, these tools are capable of harvesting data more thoroughly and expeditiously than if done manually.
Creating and preserving exactness among multiple sources over extended periods necessitates a structured system for collecting and archiving data. By developing standards for categorizing different types of information – such as timestamps, locations, or file formats – organizations can create consistency throughout their datasets which makes them easier to analyze later on down the line. It’s also beneficial to keep track of changes made along the way so that all stakeholders involved have access to updated versions if needed in future projects or reports.
Ultimately, following best practices when sourcing data helps organizations maximize their investments in their projects while minimizing potential risks associated with inaccurate or unreliable datasets. By taking proactive steps such as establishing clear goals and objectives upfront; utilizing automation tools; adopting a systematic approach; companies can ensure they have access to good data sources that are up-to-date with current trends in their industry so they don't get left behind.
Key Takeaway: Data acquisition is a must for any fruitful data science or analytics initiative, and it's best to employ good practices in order to guarantee accuracy and dependability. Automation tools such as web crawlers can help streamline the process by quickly harvesting large amounts of information with precision while developing standards for categorizing different types of data will create consistency throughout datasets over long periods of time. By following these steps proactively, organizations can maximize their investments in projects while minimizing risks associated with inaccurate sources.
FAQs in Relation to What is Data Sourcing
What is data sourcing?
This includes collecting information from databases, websites, APIs (application programming interfaces), and other online resources. Data sourcing can also involve extracting data manually or using automated tools such as web scraping to collect unstructured data. Businesses can gain advantageous knowledge by utilizing various data acquisition techniques that would be inaccessible with conventional methods.
What is an example of a data source?
A data source is any location or repository from which data can be obtained. Examples of file data sources include web APIs, databases, text files, CSV files, spreadsheets, and other structured documents such as XML and JSON. Data sources may also come from online services like social media platforms or external third-party applications that provide access to information. Furthermore, various tools can be employed to draw out unstructured data, for instance, web scraping technology which allows users to obtain a large quantity of pertinent information quickly and effortlessly.
1. Web APIs:
Web APIs are a great source of data for web extraction projects as they provide access to structured and unstructured data from websites, databases, and other sources in an organized manner.
2. HTMLs:
By leveraging HTML scraping, it is possible to gather specific pieces of information or entire datasets from web pages. It can be used to extract specific pieces of information or entire datasets from web pages.
3. Databases:
Databases are a popular source of data, thanks to their capacity for keeping vast amounts of structured information in an orderly fashion that can be readily examined and analyzed.
4. Social Media Data:
Social media platforms like Twitter, Facebook, Instagram, etc., offer up valuable insights into consumer behavior which can be leveraged through web extraction techniques such as API calls or text mining algorithms applied directly on social media posts/comments/reviews, etc.
5 File Downloads:
Files can be easily acquired from the web with specialized tools which enable rapid downloading of extensive amounts of unprocessed information without having to manually search each page or create database inquiries.
What are the types of data sources?
Data sources can be divided into two main categories: structured and unstructured. Structured data sources refer to those which possess a predetermined format, such as databases, spreadsheets or other forms of organized storage. Unstructured data sources include webpages, emails, images, videos and audio files which do not have any specific structure or organization. Data scientists and analysts use various techniques to extract meaningful information from these different types of data sources in order to gain insights for their projects.
Conclusion
With careful planning and thoughtful execution, it's possible to source quality datasets that enable meaningful insights into complex problems. By following best practices such as researching potential sources thoroughly before beginning extraction work or validating accuracy after acquisition, organizations can ensure their success in collecting valuable data sets through effective data-sourcing processes.
Let us help you unlock the potential of your data with our comprehensive web data extraction solutions. Our team of experts can provide tailored strategies to ensure that you get the most out of your online resources.